BCA 3rd Year Data Warehousing Notes Study Material
Chapter Wise BCA 3rd Year Data Warehousing Notes Study Material: If you choose to do a Bachelor of Computer Application, it is BCA. BCA is a three-year program in most universities. After getting enrolled for BCA, There are certain things you require the most to get better grades/marks in BCA. Out of those, there are BCA Notes and BCA Semester Wise Study Material. BCA Question Answers along with BCA Previous Year Papers. At gurujistudy.com you can easily get all these study materials and notes for free.
If you are a BCA Student there is a single-stop destination as far as preparation for BCA Examination is concerned. Here in this post, we are happy to provide you with Topic Wise & Chapter Wise BCA 3rd Year Data Warehousing Notes Study Material.
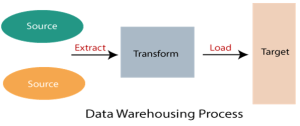
BCA 3rd Year Data Warehousing Notes Study Material
Understanding Data Warehouse
- The Data Warehouse is that database which is kept separate from the organization’s operational database.
- There is no frequent updation done in data warehouse.
- Data warehouse possess consolidated historical data which helps the organization to analyse it’s business.
- Data warehouse helps the executives to organize, understand and use their data to take strategic decision.
- Data warehouse systems available which helps in integration of diversity of application systems.
- The Data warehouse system allows analysis of consolidated historical data analysis.
Definition
- Data warehouse is subject oriented, integrated, time-variant and non-volatile collection of data that support management’s decision-making process.
- Data warehousing is the process of constructing and using the data warehouse. The data warehouse is constructed by integrating the data from multiple heterogeneous sources. This data warehouse supports analytical reporting, structured and/or ad hoc queries and decision making. Data warehousing involves data cleaning, data integration and data consolidations. (BCA 3rd Year Data Warehousing Notes Study Material)
- A data warehouse is a collection of data marts representing historical data from different operations in the company. This data is stored in a structure optimized for querying and data analysis as a data warehouse. Table design, dimensions and organization should be consistent throughout a data warehouse so that reports or queries across the data warehouse are consistent. A data warehouse can also he viewed as a database for historical data from different functions within a company.
- The term Data Warehouse was coined by Bill Inmon in 1990, which he defined in the following way: “A warehouse is a subject-oriented, integrated, time-variant and non-volatile collection of data in support of management’s decision-making process”. (BCA 3rd Year Data Warehousing Notes Study Material)
Definition
He defined the terms in the sentence as follows:
Subject Oriented: Data that gives information about a particular subject instead of about a company’s ongoing operations.
Integrated: Data that is gathered into the data warehouse from a variety of sources and merged into a coherent whole.
Time-variant: All data in the data warehouse is identified with a particular time period.
Non-volatile: Data is stable in a data warehouse. More data is added but data is never removed.
This enables management to gain a consistent picture of the business. It is a single, complete and consistent store of data obtained from a variety of different sources made available to end users in what they can understand and use in a business context. It can be:
- Used for decision Support.
- Used to manage and control business.
- Used by managers and end-users to understand the business and make judgements.
Data warehousing is an architectural construct of information systems that provides users with current and historical decision support information that is hard to access or present in traditional operational data stores. (BCA 3rd Year Data Warehousing Notes Study Material)
-
Difference between Data Warehouse and Operational Databases
The following are the reasons why data warehouse are kept separate from operational
databases:
- The operational database is constructed for well. known tasks and workload such as searching particular records, indexing, etc. but the data warehouse queries are often complex and it presents the general form of data.
- Operational databases supports the concurrent processing of multiple transactions. Concurrency control and recovery mechanism are required for operational databases to ensure robustness and consistency of database. (BCA 3rd Year Data Warehousing Notes Study Material)
- Operational database query allow to read, modify operations while the OLAP query need only read only access of stored data.
- Operational database maintain the current data on the other hand data warehouse maintain the historical data.
Data Warehouse Features
The key features of data warehouse such as subject oriented, integrated, non-volatile and time-variant are discussed below:
- Subject Oriented: The data warehouse is subject oriented because it provides us the information around a subject rather the organization’s ongoing operations. These subjects can be product, customers, suppliers, sales, revenue etc. The data warehouse does not focus on the ongoing operations rather it focuses on modelling and analysis of data for decision making.
- Integrated: Data warehouse is constructed by integration of data from heterogeneous sources such as relational databases, flat files etc. This integration enhance the effective analysis of data. (BCA 3rd Year Data Warehousing Notes Study Material)
- Time-Variant: The data in data warehouse is identified with a particular time period. The data in data warehouse provides information from historical point of view.
- Non-Volatile: Non-volatile means that the previous data is not removed when new data is added to it. The data warehouse is kept separate from the operational database therefore frequent changes in operational database is not reflected in data warehouse.
Note: Data warehouse does not require transaction processing, recovery and concurrency control because it is physically stored separate from the operational database.
- Metadata: Metadata is simply defined as data about data. The data that are used to represent other data is known as metadata. For example, the index of a book serve as metadata for the contents in the book. In other words, we can say that metadata is the summarized data that lead us to the detailed data.
In terms of data warehouse we can define metadata as following:
- Metadata is a road map to data warehouse.
- Metadata in data warehouse define the warehouse objects.
- The metadata act as a directory. This directory helps the decision support system to locate the contents of data warehouse.
III. Metadata Respiratory
The metadata respiratory is an integral part of data warehouse system. The metadata respiratory contains the following metadata:
- Business Metadata: This metadata has the data ownership information, business definition and changing policies.
- Operational Metadata: This metadata includes currency of data and data lineage. Currency of data means whether data is active, archived or purged. Lineage of data means history of data migrated and transformation applied on it. (BCA 3rd Year Data Warehousing Notes Study Material)
- Data for mapping from operational environment to data warehouse: This metadata includes source databases and their contents, data extraction, data partition, cleaning, transformation rules, data refresh and purging rules. (BCA 3rd Year Data Warehousing Notes Study Material)
- The algorithms for summarization: This includes dimension algorithms, data on granularity, aggregation, summarizing, etc.
Data Warehouse Applications
Data warehouse is widely used in the following fields:
- Financial services
- Banking services
- Consumer goods
- Retail sectors
- Controlled manufacturing
Its Types
Information processing, analytical processing and data mining are the three types of data warehouse applications that are discussed below:
- Information processing: Data warehouse allows us to process the information stored in it. The information can be processed by means of querying, basic statistical analysis, reporting using crosstabs, tables, charts, or graphs. (BCA 3rd Year Data Warehousing Notes Study Material)
- Analytical processing: Data warehouse supports analytical processing of the information stored in it. The data can be analysed by means of basic OLAP operations, including slice-and-dice, drill down, drill up, and pivoting. (BCA 3rd Year Data Warehousing Notes Study Material)
- Data mining: Data mining supports knowledge discovery by finding the hidden patterns and associations, constructing analytical models, performing classification and prediction. These mining results can be presented using the visualization tools. (BCA 3rd Year Data Warehousing Notes Study Material)
Using Data Warehouse Information
There are decision support technologies available which help to utilize the data warehouse. These technologies help the executives to use the warehouse quickly and effectively. They can gather the data, analyse it and take the decisions based on the information in the warehouse. The information gathered from the warehouse can be used in any of the following domains:
- Tuning production strategies: The product strategies can be well tuned by repositioning the products and managing product portfolios by comparing the sales quarterly or yearly.
- Customer analysis: The customer analysis is done by analyzing the customer’s buying preferences, buying time, budget cycles etc.
- Operations analysis: Data warehousing also helps in customer relationship management, making environmental corrections. The Information also allow us to analyse the business operations. (BCA 3rd Year Data Warehousing Notes Study Material)
VII. Benefits of Data Warehousing
- Data warehouses are designed to perform well with aggregate queries running on large amounts of data.
- The structure of data warehouses is easier for end users to navigate, understand and query against unlike the relational databases primarily designed to handle lots of transactions. (BCA 3rd Year Data Warehousing Notes Study Material)
- Data warehouses enable queries that cut across different segments of a company’s operation e.g., production data could be compared against inventory data even if they were originally stored in different databases with different structures. (BCA 3rd Year Data Warehousing Notes Study Material)
- Queries that would be complex in very normalized databases could be easier to build and maintain in data warehouses, decreasing the workload on transaction systems.
- Data warehousing is an efficient way to manage and report on data that is from a variety of sources, non-uniform and scattered throughout a company.
- Data warehousing is an efficient way to manage demand for lots of information from lots of users.
- Data warehousing provides the capability to analyze large amounts of historical data for nuggets of wisdom that can provide an organization with competitive advantage.
VIII. Data Warehouse Tools and Utilities Functions
The followings are the functions of data warehouse tools and utilities:
- Data extraction: Data extraction involves gathering the data from multiple heterogeneous sources.
- Data cleaning: Data cleaning involves finding and correcting the errors in data.
- Data transformation: Data transformation involves converting data from legacy format to warehouse format.
- Data loading: Data loading involves sorting, summarizing, consolidating, checking integrity and building indices and partitions.
- Refreshing: Refreshing involves updating from data sources to warehouse.
Note: Data Cleaning and Data Transformation are important steps in improving the quality of data and data mining results.
-
Data Mart
Data mart contains the subset of organisation-wide data. This subset of data is valuable to specific group of an organisation. In other words, we can say that data mart contains only that data which is specific to a particular group. For example, the marketing data mart may contain only data related to item, customers and sales. The data mart is confined to subjects. (BCA 3rd Year Data Warehousing Notes Study Material)
Points to remember about data mart:
- window based or Unix/Linux based servers are used to implement data mart. They are implemented on low-cost server.
- The implementation cycle of data mart is measured in short period of time i.e. in weeks rather than months or years.
- The life cycle of a data mart may be complex in long run. if it’s planning and design are not organisation-wide.
- Data mart is customized by department.
- Data mart is small in size.
- The source of data mart is departmentally structured data warehouse.
- Data mart is flexible.
Need to create data mart:
The followings are the reasons to create data mart:
- To partition data in order to impose access control strategies.
- To speed up the queries by reducing the volume of data to be scanned.
- To segment data into different hardware platforms.
- To structure data in a form suitable for a user access tool.
Note: Do not data mart for any other reason since the operation cost of data marting could be very high. Before data marting, make sure that data marting strategy is appropriate for your particular solution. (BCA 3rd Year Data Warehousing Notes Study Material)
Steps to determine that data mart appears to fit the bill:
Following steps need to be followed to make cost effective data marting:
- Identify the functional splits
- Identify user access tool requirements
- Identify access control issues
Data Warehouse Design Approaches
Data warehouse design is one of the key technique in building the data warehouse. Choosing a right data warehouse design can save the project time and cost. Basically, there are two data warehouse design approaches are popular. (BCA 3rd Year Data Warehousing Notes Study Material)
-
Top-Down Design
In the top-down design approach the, data warehouse is built first. The data marts are then created from the data warehouse.
If you use a top-down approach, you will have to analyze global business needs, plan how to develop a data warehouse, design it, and implement it as a whole. This procedure is promising: it will achieve excellent results because it is based on a global picture of the goal to achieve, and in principle it ensures consistent, well integrated data warehouses. (BCA 3rd Year Data Warehousing Notes Study Material)
However, a long story of failure with top-down approaches teaches that:
- High-cost estimates with long-term implementations discourage company managers from embarking on these kind of projects;
- Analyzing and bringing together all relevant sources is a very difficult task, also because it is not very likely that they are all available and stable at the same time;
- It is extremely difficult to forecast the specific needs of every department involved in a project, which can result in the analysis process coming to a standstill;
- Since, no prototype is going to be delivered in the short term, users cannot check for this project to be useful, so they lose trust and interest in it.
(i) Advantages of top-down design are
- Provides consistent dimensional views of data across data marts, as all data marts are loaded from the data warehouse.
- This approach is robust against business changes. Creating a new data mart from the data warehouse is very easy.
(ii) Disadvantages of top-down design are:
- This methodology is inflexible to changing departmental needs during implementation phase.
- It represents a very large project and the cost of implementing the project is significant.
Bottom-Up Design
In the bottom-up design approach, the data marts are created first to provide reporting capability. A data mart addresses a single business area such as sales, finance etc. These data marts are then integrated to build a complete data warehouse. The integration of data marts is implemented using data warehouse bus architecture. In the bus architecture, a dimension is shared between facts in two or more data marts. These dimensions are called conformed dimensions. These conformed dimensions are integrated from data marts and then data warehouse is built. (BCA 3rd Year Data Warehousing Notes Study Material)
In a bottom-up approach, data warehouses are incrementally built and several data marts are iteratively created. Each data mart is based on a set of facts that are linked to a specific company department and that can be interesting for a user subgroup (for example, data marts for inventories, marketing, and so on). If this approach is coupled with quick prototyping, the time and cost needed for implementation can be reduced so remarkably that company managers will notice how useful the project being carried out is. In this way, that project will still be of interest. (BCA 3rd Year Data Warehousing Notes Study Material)
Bottom-Up Design
The bottom-up approach turns out to be more cautious than the top-down one and it is almost universally accepted. Naturally the bottom-up approach is not risk-free, because it gets a partial picture of the whole field of application. We need to pay attention to the first data mart to be used as prototype to get the best results: this should play a very strategic role in a company. In fact, its role is so crucial that this data mart should be a reference point for the whole data warehouse. In this way, the following data marts can be easily added to the original one. Moreover, it is highly advisable that the selected data mart exploit consistent data already made available.
(i) Advantages of bottom-up design are
- This model contains consistent data marts and these data marts can be delivered quickly.
- As the data marts are created first, reports can be generated quickly.
- The data warehouse can be extended easily to accommodate new business units. It is just creating new data marts and then integrating with other data marts.
(ii) Disadvantages of bottom-up design are
The positions of the data warehouse and the data marts are reversed in the bottom-up approach design.
Identify The Functional Splits
In this step, we determine that whether the natural functional split is there in the organization. We look for departmental splits and we determine whether the way in which department use information tends to be in isolation from the rest of the organization. Let’s have an example…
Suppose in a retail organization where the each merchant is accountable for maximizing the sales of a group of products. For this the information that is valuable is:
- sales transaction on daily basis
- sales forecast on weekly basis
- stock position on daily basis
- stock movements on daily basis
As the merchant is not interested in the products they are not dealing with, so the data marting is subset of the data dealing which the product group of interest. Following diagram shows data marting for different users. (BCA 3rd Year Data Warehousing Notes Study Material)
Issues in determining the functional split:
- The structure of the department may change.
- The products might switch from one department to other.
- The merchant could query the sales trend of other products to analyse what is happening to the sales.
These are issues that need to be taken into account while determining the functional split.
Note: We need to determine the business benefits and technical feasibility of using data mart.
Identify User Access Tool Requirements
For the user access tools that require the internal data structures we need data mart to Support such tools. The data in such structures are outside the control of data warehouse but need to be populated and updated on regular basis. (BCA 3rd Year Data Warehousing Notes Study Material)
There are some tools that populated directly from the source system but some can not. Therefore additional requirements outside the scope of the tool are needed to be identified for future. (BCA 3rd Year Data Warehousing Notes Study Material)
Note: In order to ensure consistency of data across all access tools the data should not be directly populated from the data warehouse rather each tool must have its own data mart. (BCA 3rd Year Data Warehousing Notes Study Material)
Identify Access Control Issues
There need to be privacy rules to ensure the data is accessed by the authorised users only. For example, in data warehouse for retail banking institution ensure that all the accounts belong to the same legal entity. Privacy laws can force you to totally prevent access to information that is not owned by the specific bank.
Data mart allows us to build complete wall by physically separating data segments within the data warehouse. To avoid possible privacy problems the detailed data can be removed from the data warehouse. We can create data mart for each legal entity and load it via data warehouse, with detailed account data.
- Designing Data Mart
The data mart should be designed as smaller version of starflake schema within the data warehouse and should match to the database design of the data warehouse. This helps in maintaining control on database instances. (BCA 3rd Year Data Warehousing Notes Study Material)
The summaries are data marted in the same way as they would have been designed within the data warehouse. Summary tables help to utilize all dimension data in the starflake schema. (BCA 3rd Year Data Warehousing Notes Study Material)
- Cost of Data Marting
The followings are the cost measures for data marting:
- Hardware and software cost
- Network access
- Time window constraints
(i) Hardware and Software Cost
Although the data mart are created on the same hardware even then they require some additional hardware and software. To handle the user queries there is need of additional processing power and disk storage. If the detailed data and the data mart exist within the data warehouse then we would face additional cost to store and manage replicated data. (BCA 3rd Year Data Warehousing Notes Study Material)
Note: The data marting is more expensive than aggregations therefore it should be used as an additional strategy not as an alternative strategy.
(ii) Network Access
The data mart could be on different locations from the data warehouse so we should ensure that the LAN or WAN has the capacity to handle the data volumes being transferred within the data mart load process. (BCA 3rd Year Data Warehousing Notes Study Material)
(iii) Time Window Constraints
The extent to which the data mart loading process will eat into the available time window will depend on the complexity of the transformations and the data volumes being shipped. Feasiblity of number of data mart depends on:
- Network capacity
- Time window available
- Volume of data being transferred
- Mechanisms being used to insert data into data mart
Three-Tier Data Warehouse Architecture
Generally the data warehouses adopt the three-tier architecture. Followings are the three tiers of data warehouse architecture:
- Bottom tier: The bottom tier of the architecture is the data warehouse database server. It is the relational database system. We use the back end tools and utilities to feed data into bottom tier these back end tools and utilities performs the Extract, Clean, Load, and refresh functions.
- Middle tier: In the middle tier, we have OLAP Server. the OLAP Server can be implemented in either of the following ways:
(i) By relational OLAP (ROLAP), which is an extended relational database management system. The ROLAP maps the operations on multidimensional data to standard relational operations. (BCA 3rd Year Data Warehousing Notes Study Material)
(ii) By Multidimensional OLAP (MOLAP) model, which directly implements multidimensional data and operations.
- Top tier: This tier is the front-end client layer. This layer holds the query tools and reporting tools, analysis tools and data mining tools.
Following diagram explains the three-tier architecture of data warehouse:
Data Warehouse Models
From the perspective of data warehouse architecture we have the following data warehouse models:
- Virtual warehouse
- Data mart
- Enterprise warehouse
Virtual Warehouse
- The view over a operational data warehouse is known as virtual warehouse. It is easy to built the virtual warehouse.
- Building the virtual warehouse requires excess capacity on operational database servers.
Data Mart
- Data mart contains the subset of organisation-wide data.
- This subset of data is valuable to specific group of an organisation.
Note: In other words, we can say that data mart contains only that data which is specific to a particular group. For example, the marketing data mart may contain only data related to item, customers and sales. The data mart are confined to subjects. (BCA 3rd Year Data Warehousing Notes Study Material)
III. Enterprise Warehouse
- The enterprise warehouse collects all the information all the subjects spanning the entire organization.
- This provide us the enterprise-wide data integration.
- This provide us the enterprise-wide data integration.
- The data is integrated from operational systems and external information providers.
- This information can vary from a few gigabytes to hundreds of gigabytes, terabytes or beyond.
Load Manager
- This component performs the operations required to extract and load process.
- The size and complexity of load manager varies between specific solutions from data warehouse to data warehouse.
Load Manager Architecture
The load manager performs the following functions:
- Extract the data from source system.
- Fast Load the extracted data into temporary data store.
- Perform simple transformations into structure similar to the one in the data warehouse.
Extract Data From Source
The data is extracted from the operational databases or the external information providers. Gateways is the application programs that are used to extract data. It is supported by underlying DBMS and allows client program to generate SQL to be executed at a server. Open Database Connection (ODBC), Java Database Connection (JDBC), are examples of gateway. (BCA 3rd Year Data Warehousing Notes Study Material)
Fast Load
- In order to minimize the total load window the data need to be loaded into the warehouse in the fastest possible time.
- The transformations affects the speed of data processing. (BCA 3rd Year Data Warehousing Notes Study Material)
- It is more effective to load the data into relational database prior to applying transformations and checks.
- Gateway technology proves to be not suitable, since they tend not be performance when large data volumes are involved.
Simple Transformations
While loading it may be required to perform simple transformations. After this has been completed we are in position to do the complex checks. Suppose we are loading the EPOS sales transaction we need to perform the following checks:
- Strip out all the columns that are not required within the warehouse.
- Convert all the values to required data types.
Warehouse Manager
Warehouse manager is responsible for the warehouse management process.
The warehouse manager consist of third party system software, C programs and shell scripts.
The size and complexity of warehouse manager varies between specific solutions.
- Warehouse Manager Architecture
The warehouse manager includes the following:
- The Controlling process
- Stored procedures or C with SQL
- SQL Scripts
- Backup/Recovery tool
2. Operations Performed By Warehouse Manager
- Warehouse manager analyses the data to perform consistency and referential integrity checks.
- Creates the indexes, business views, partition views against the base data.
- Generates the new aggregations and also updates the existing aggregation. Generates the normalizations.
- Warehouse manager transforms and merge the source data into the temporary store into the published data warehouse.
- Backup the data in the data warehouse. (BCA 3rd Year Data Warehousing Notes Study Material)
- Warehouse manager archives the data that has reached the end of its captured life.
Note: Warehouse manager also analyses query profiles to determine index and aggregations are appropriate.
Query Manager
- Query manager is responsible for directing the queries to the suitable tables.
- By directing the queries to appropriate table the query request and response process is speed up.
- Query manager is responsible for scheduling the execution of the queries posed by the user.
1. Query Manager Architecture
Query manager includes the following:
- The query redirection via C tool or RDBMS.
- Stored procedures.
- Query management tool.
- Query scheduling via C tool or RDBMS.
- Query scheduling via third party software.
II, Detailed Information
The following diagram shows the detailed information:
The detailed information is not kept online rather is aggregated to the next level of detail and then archived to the tape. The detailed information part of data warehouse keep the detailed information in the starflake schema. the detailed information is loaded into the data warehouse to supplement the aggregated data.
Note: If the detailed information is held offline to minimize the disk storage we should make sure that the data has been extracted, cleaned up, and transformed then into starflake schema before it is archived. (BCA 3rd Year Data Warehousing Notes Study Material)
III. Summary Information
- In this area of data warehouse the predefined aggregations are kept.
- These aggregations are generated by warehouse manager.
- This area changes on ongoing basis in order to respond to the changing query profiles.
- This area of data warehouse must be treated as transient.
Points to remember about summary information:
- The summary data speed up the performance of common queries.
- It increases the operational cost.
- It need to be updated whenever new data is loaded into the data warehouse.
- It may not have been backed up, since it can be generated fresh from the detailed information.
Future Aspects of Data Warehousing
Following are the future aspects of Data Warehousing:
- As we have seen that the size of the open database has grown approximately double the magnitude in last few years. This change in magnitude is of greater significance.
- As the size of the databases grow, the estimates of what constitutes a very large database continues to grow. (BCA 3rd Year Data Warehousing Notes Study Material)
- The Hardware and software that are available today do not allow to keep a large amount of data online. For example a Telco call record require 10TB of data to be kept online which is just a size of one month record. If It require to keep record of sales, marketing customer, employee etc. then the size will be more than 100 TB.
- The record not only contain the textual information but also contain some multimedia data. Multimedia data cannot be easily manipulated as text data. Searching the multimedia data is not an easy task whereas the textual information can be retrieved by the relational software available today.
- Apart from size planning, building and running ever-larger data warehouse systems are very complex. As the number of users increases the size of the data warehouse also increases. These users will also require to access to the system. (BCA 3rd Year Data Warehousing Notes Study Material)
- With growth of internet, there is requirement of users to access data online.
BCA 3rd Year Data Warehousing Notes Study Material
BCA 3rd Year Data Warehousing Notes Study Material
Chapter Wise BCA 3rd Year Data Warehousing Notes Study Material
Chapter Wise BCA 3rd Year Data Warehousing Notes Study Material
BCA 3rd Year Data Warehousing Notes Study Material
BCA 3rd Year Data Warehousing Notes Study Material
Chapter Wise BCA Data Warehousing Notes Study Material
Chapter Wise BCA Data Warehousing Notes Study Material
BCA 3rd Year Data Warehousing Notes Study Material
BCA 3rd Year Data Warehousing Notes Study Material
Chapter Wise BCA Data Warehousing Notes Study Material
Chapter Wise BCA Data Warehousing Notes Study Material